Best Practices for Testing AI Applications
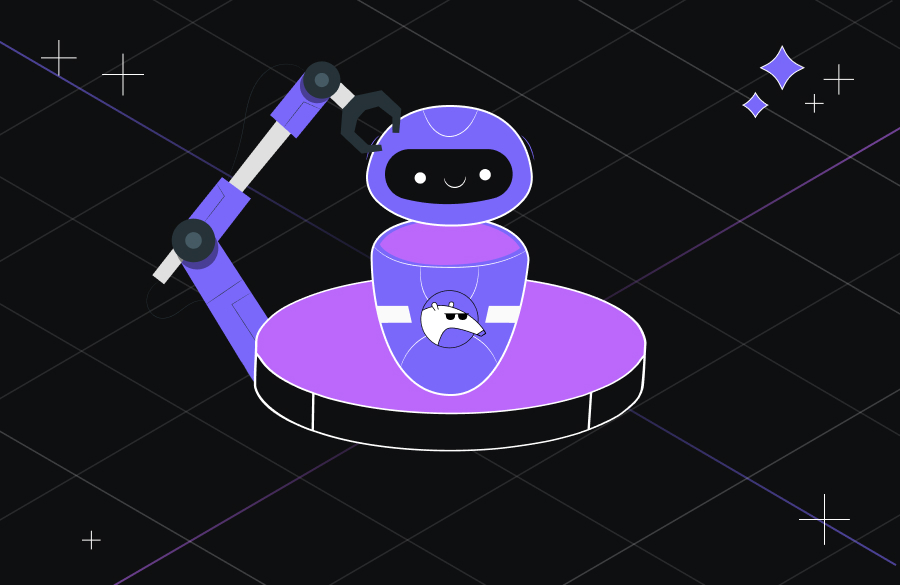
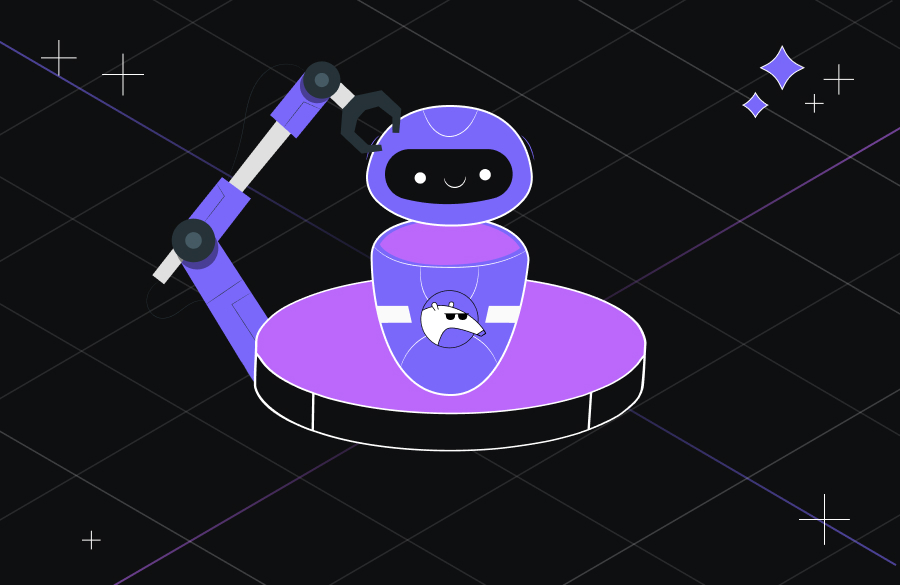
Artificial intelligence (AI) and its applications play a crucial role in the modern technological landscape. AI can now handle many human tasks in various industries, such as healthcare, finance, and manufacturing. As industries have adapted and integrated AI into their day-to-day operations, ensuring these AI systems are functioning properly and ethically without mistakes is crucial. Hence, proper testing mechanisms must be integrated to test AI systems.
This article highlights essential best practices for testing AI applications, details why such testing is crucial, the key challenges involved, and how to establish effective testing frameworks for testing AI systems.
Why Perform AI Applications Testing?
In contrast with traditional software, AI systems learn from data, evolve, handle increasing complexity, and make decisions to different degrees of autonomy. These characteristics create several compelling reasons for rigorous testing:
1. Ensuring accuracy and reliability
- Validates consistent and accurate results across various scenarios.
- Identifies performance gaps and edge cases before deployment.
- Ensures AI can handle real-world conditions reliably.
- Maintains performance over time as data distributions change.
2. Mitigating bias and ensuring fairness
- Identifies biases that may exist in training data.
- Ensures equitable treatment across different demographic groups.
- Reveals potential impacts on users.
3. Building trust with users and stakeholders
- Demonstrates commitment to quality and responsible AI development.
- Increases the likelihood of user adoption through proven reliability.
- Builds confidence that systems will perform as expected.
- Creates transparency around how edge cases are handled.
4. Regulatory compliance
- Governments worldwide are implementing regulations governing AI use.
- AI in testing software ensures compliance with evolving regulatory requirements.
- Helps organizations avoid penalties and reputational damage.
- Regulations often mandate transparency, explainability, and fairness-all requiring thorough testing.
Key Challenges in Testing AI Applications
Testing AI applications presents unique challenges that distinguish it from traditional software testing:
1. Non-deterministic behavior
- Unlike traditional software, AI systems may generate different results for the same input.
- Variations based on training data, learning algorithms, or random initialization.
- Makes establishing clear pass/fail criteria difficult.
- Complicates reproducing issues.
2. Black-Box nature
- Many AI models operate as black boxes, and explaining their behaviors and decision-making processes is difficult.
- Lack of transparency complicates the validation of results.
3. Data dependencies
- Data quality, representativeness, and biases significantly impact performance.
- Requires evaluation with diverse, real-world data.
4. Evolving behavior
- Performance may change over time as the system learns.
- Many AI systems continue to learn and adapt after deployment.
5. Explainability requirements
- Modern regulations increasingly require AI decisions to be explainable.
- Testing must verify that AI systems can provide understandable explanations.
- Explanations must justify choices in ways humans can comprehend.
Testing AI Applications Best Practices
Implementing the following best practices can help address the challenges associated with AI application testing:
1. Define clear testing objectives
Begin by clearly defining what aspects of the AI system you need to test. These might include:
- Functional correctness: Does the AI system produce the expected outputs for given inputs?
- Performance: Does the system meet speed and resource efficiency requirements?
- Robustness: How does the system handle adversarial inputs or edge cases?
- Fairness: Does the system exhibit bias against certain groups?
- Explainability: Can the system’s decisions be understood and explained?
Each testing objective should have measurable criteria for success, even for subjective aspects like fairness and explainability.
2. Implement comprehensive data testing
Since AI systems are highly data-dependent, testing should include a thorough evaluation of training and testing data:
- Data quality assessment: Check for missing values, outliers, and inconsistencies.
- Representative sampling: Ensure test data covers the full range of scenarios the system will encounter.
- Bias detection: Analyze data for potential biases related to protected attributes.
- Data augmentation: Create synthetic data to test edge cases and underrepresented scenarios.
- Data versioning: Maintain version control for data sets to ensure reproducibility.
3. Adopt model-specific testing approaches
Different AI models require different testing approaches:
- For supervised learning models: Compare predictions against ground truth labels using appropriate metrics (accuracy, precision, recall, F1 score).
- For unsupervised learning models: Evaluate the effectiveness of cluster quality, dimensionality reduction, or anomaly detection accuracy.
- For reinforcement learning models: Test decision-making in simulated environments across various scenarios.
- For NLP models: Test linguistic capabilities, bias, and contextual understanding.
- For computer vision models: Test object recognition, scene understanding, and robustness to visual perturbations.
4. Implement adversarial testing
Adversarial testing intentionally attempts to “break” the AI system by providing challenging inputs:
- Generate adversarial examples that slightly modify inputs to cause misclassifications.
- Test boundary conditions and edge cases that might confuse the system.
- Evaluate the system’s robustness to noisy or corrupted inputs.
- Test the system with out-of-distribution data to assess generalization capabilities.
5. Conduct A/B testing
When deploying new AI models or updating existing ones:
- Run the new and old versions in parallel to compare performance.
- Gradually roll out changes to limit potential negative impacts.
- Collect user feedback to evaluate subjective aspects of performance.
- Monitor key metrics to detect unexpected behavior changes.
6. Establish continuous testing workflows
AI testing should be continuous throughout the application lifecycle:
- Implement automated testing pipelines that run whenever models or data change.
- Monitor deployed models for performance drift over time.
- Retrain and retest models periodically with fresh data.
- Implement feedback loops to capture real-world performance issues.
7. Ensure explainability and interpretability
Make AI decision-making more transparent:
- Use interpretable models when possible (decision trees, linear models).
- Apply post hoc explanation techniques for complex models (LIME, SHAP values).
- Verify that explanations are consistent, accurate, and understandable to users.
- Test the system’s ability to provide explanations for specific decisions.
AI-Driven Strategies for Software Testing
AI itself can enhance testing processes for both traditional software and AI applications:
1. Automated test generation
- AI can analyze application code or specifications to automatically generate test cases.
- Improves test coverage and identifies edge cases human testers might miss.
- Generates new tests targeting untested functionality.
2. Intelligent test prioritization
- AI can prioritize test cases based on the likelihood of finding defects.
- Considers recent code changes or historical failure patterns.
3. Visual user interface (UI) testing
- AI-powered visual testing tools detect visual regressions in user interfaces.
- Uses computer vision to compare screenshots before and after changes.
- Identifies unexpected visual differences.
4. Anomaly detection in test results
- AI can identify anomalous patterns in test results or application logs.
- Helps detect subtle defects not captured by traditional pass/fail criteria.
5. Predictive analytics for quality assurance
- Machine learning models predict which components are most likely to contain defects.
- Based on code complexity, change history, and other factors.
- Helps testing teams allocate resources more effectively.
6. Continuous monitoring
- Tracks performance degradation, data drift, and other issues.
- Sends alerts when metrics deviate from expected ranges.
- Enables proactive intervention.
7. Explainability tools
- Incorporates various explainability techniques.
- Makes AI decisions more transparent.
- Helps verify that the system’s reasoning aligns with business rules and ethical guidelines.
AI Testing With Qodo
Qodo provides a comprehensive AI-driven platform that automates code reviews, test generation, and quality assurance.
Qodo offers three main products:
- Qodo Gen: An IDE plugin that generates context-aware code and tests, offers smart code suggestions, and helps developers write better tests directly within their environment.
- Qodo Cover: A CLI tool that analyzes codebases to identify test coverage gaps and automatically generates new tests to improve test effectiveness.
- Qodo Merge: A Git-integrated agent that enhances pull request workflows by reviewing code, highlighting issues, and generating AI-powered suggestions for improvements.
Here is how Qodo simplifies AI-based testing:
- A unified platform integrates multiple critical capabilities into one solution.
- Eliminates the need for multiple tools while maintaining consistent methodologies.
- Automated bias detection provides detailed metrics and mitigation strategies.
- Real-time continuous monitoring catches performance issues and data drift early.
- Built-in explainability tools ensure AI decision transparency.
- Automated compliance documentation streamlines regulatory processes.
- Intuitive visualizations make complex testing results easily accessible.
- Reduces complexity, time, and expertise needed for thorough AI testing.
Wrapping Up
As AI continues to transform industries and society, rigorous testing becomes increasingly critical to ensure these powerful systems operate reliably, fairly, and ethically. By adopting the practices described in this article, organizations can develop AI applications that build user trust and create sustainable business value by mitigating risk.
Frequently Asked Questions (FAQ)
1. How do you test for bias in AI systems?
Testing for bias involves demographic analysis across different groups and counterfactual testing with:
- Varied sensitive attributes.
- Applying fairness metrics (equal opportunity difference, disparate impact ratio).
- Analyzing training data for skewed distributions.
- Conducting intersectional analysis.
This requires diverse test data and regular retesting, as bias can emerge over time.
2. What are the best practices for performance testing of AI applications?
- Measure response times under various loads.
- Determine throughput capacity.
- Monitor resource utilization (CPU, memory, GPU).
- Verify scalability with increasing users/data.
- Test both batch and real-time performance.
- Evaluate model optimization impacts and for edge AI.
- Test on resource-constrained devices.
- Testing should occur in production-like environments.
3. What are the ethical considerations when testing AI applications?
Ensure data was collected with informed consent and protect privacy through anonymization. This should include,
- Diverse perspectives in testing teams.
- Environmental impacts of compute-intensive testing.
- Document methodologies and limitations transparently.
- Potential societal impacts.
- Compliance with relevant regulations like GDPR or CCPA.
4. How often should AI models be retested?
AI models should be retested regularly-typically monthly for actively learning systems, after any significant data updates, when deployment environments change, following model retraining, and whenever performance metrics show concerning trends. Critical applications may require continuous monitoring with automated alerts.
5. What role does explainability play in AI testing?
Explainability testing verifies that AI systems can provide understandable justifications for their decisions. This includes,
- Evaluating feature importance methods.
- Testing counterfactual explanations.
- Ensuring explanations are consistent across similar inputs.
- Confirming that explanations align with domain expertise.
This is essential for regulatory compliance and building user trust.
6. How can organizations effectively test AI systems with limited labeled data?
Organizations can address limited labeled data challenges through:
- Data augmentation techniques (generating synthetic examples).
- Transfer learning (using pre-trained models).
- Active learning (strategically selecting the most informative samples for labeling).
- Semi-supervised approaches (utilizing unlabeled data alongside limited labeled data).
- Cross-validation with small datasets (maximizing information from available labels).
- Careful evaluation of generalization using held-out test sets.
Document data limitations transparently and consider whether the available data sufficiently represents the deployment environment.
7. What are the key differences between testing traditional software and AI applications?
- Testing traditional software focuses on deterministic behaviors with well-defined inputs and expected outputs, while AI testing handles probabilistic systems where “correct” answers may vary.
- Traditional testing emphasizes functional requirements and code coverage, whereas AI testing prioritizes data quality, statistical performance, generalization capability, and bias detection.
- Traditional software has stable behavior after deployment, while AI systems may continue learning and evolving, requiring continuous monitoring and retraining processes.